Utilizing Long Short-Term Memory (LSTM) models for predicting stock prices has become a popular application of artificial intelligence and machine learning techniques in the financial sector. LSTM, a type of recurrent neural network (RNN), is well-suited for processing and predicting sequences of data, making it ideal for analyzing time-series data like stock prices. One of the main advantages of LSTM networks is their ability to capture long-term dependencies and patterns in data, which is crucial for understanding the complexities of stock market movements.
When predicting stock prices using LSTM models, historical price data is fed into the network to identify underlying patterns. The LSTM model learns from past price movements, trading volumes, and other relevant factors to make predictions about future prices. Feature engineering plays a significant role, as analysts often incorporate various technical indicators, market sentiment, and economic indicators to enhance the model’s predictive accuracy.
Data source / sample data of stock price : NFLX
Software/Programming Languages Used:
- Colab
- Python
- Excel
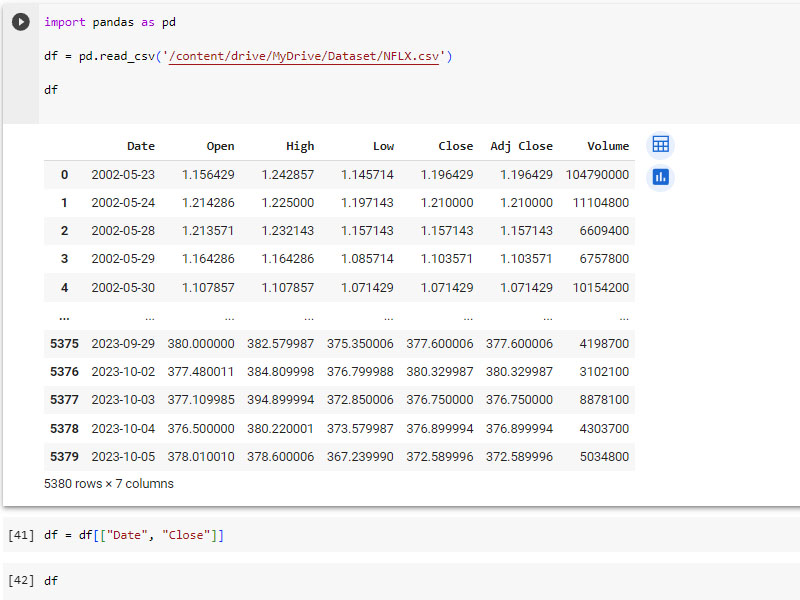
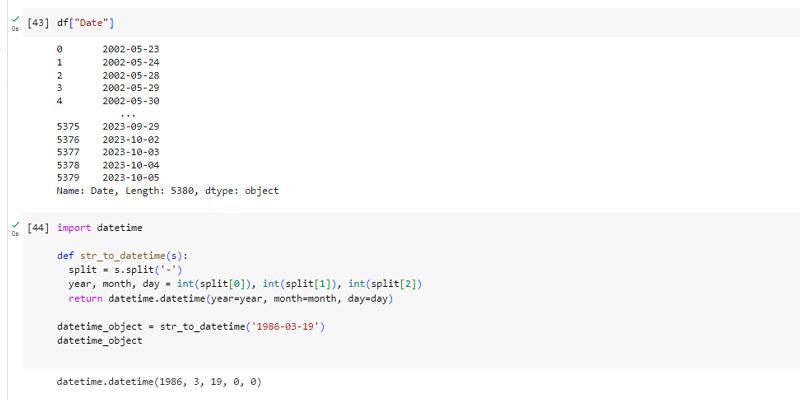
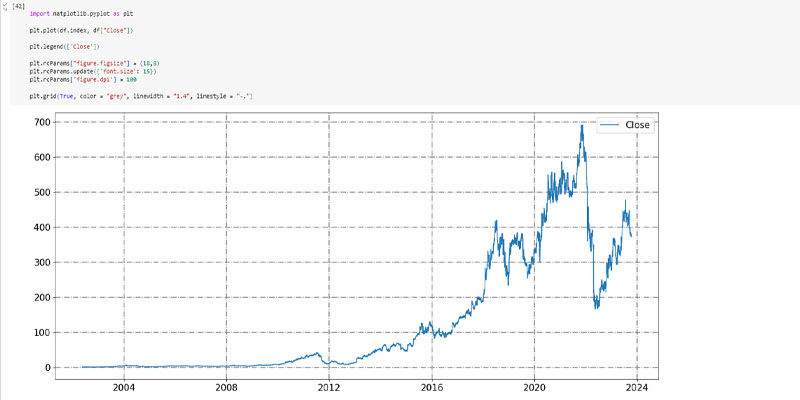
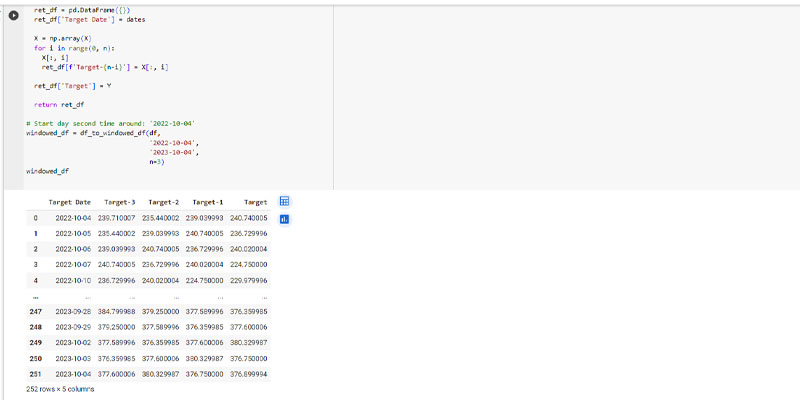
Set Target Date: 2022-10-04 to 2023-10-04
Target price: Target, Target 1, Target 2 and Target 3
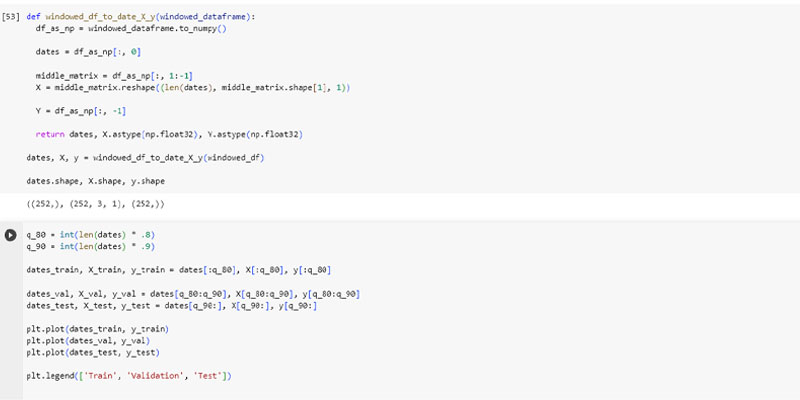
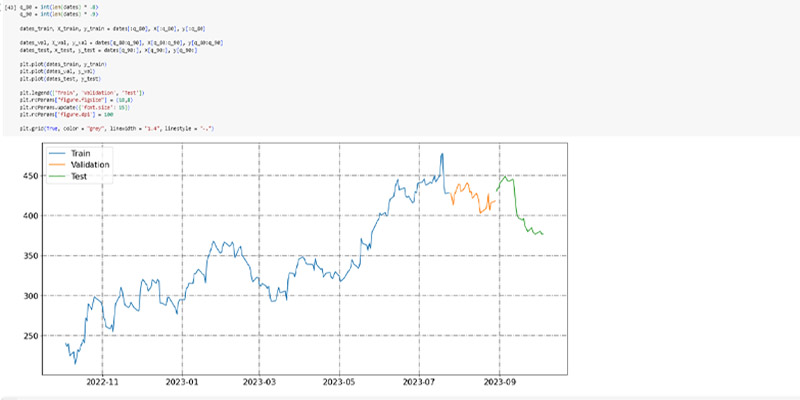
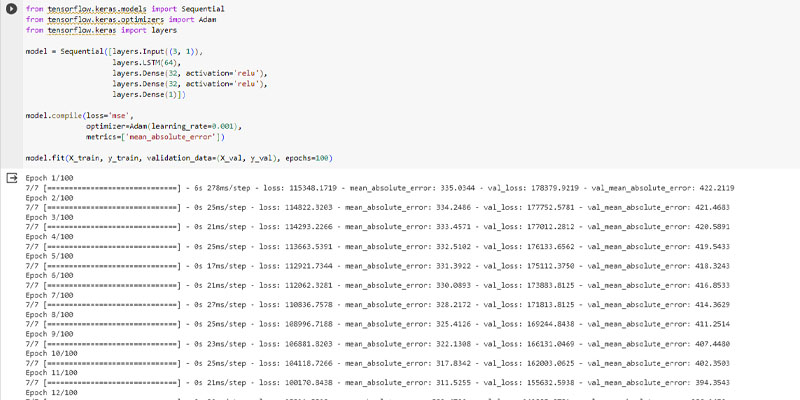
Dates Training – epochs 100 rows
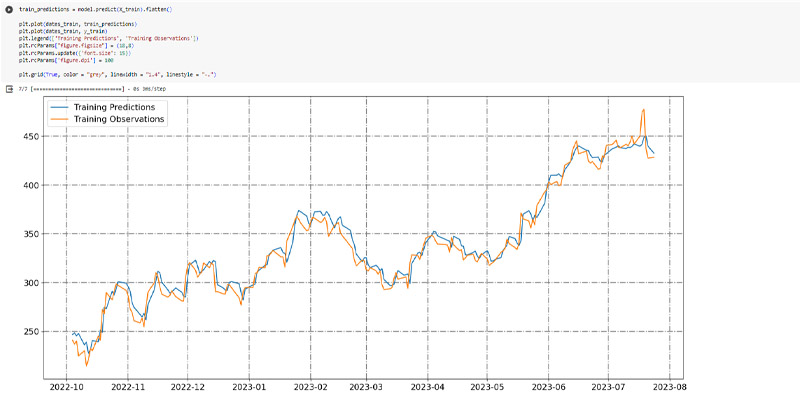
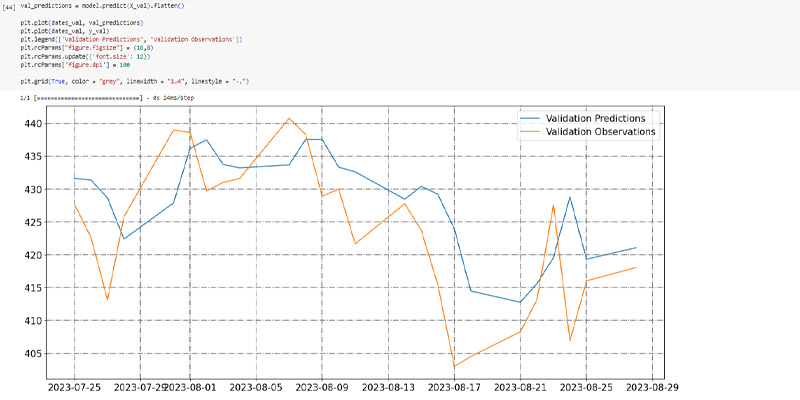
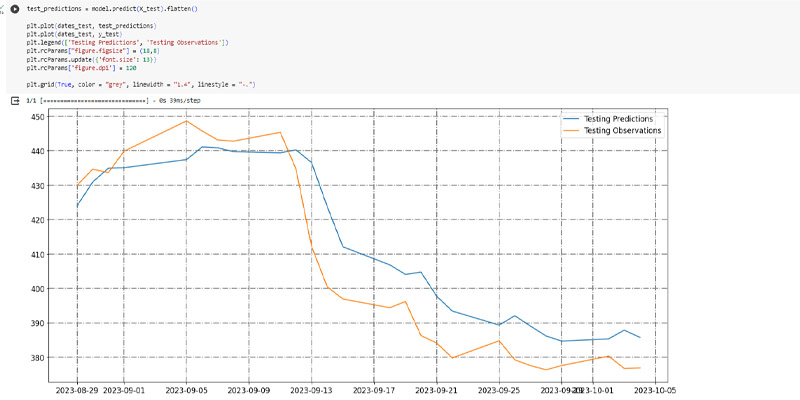
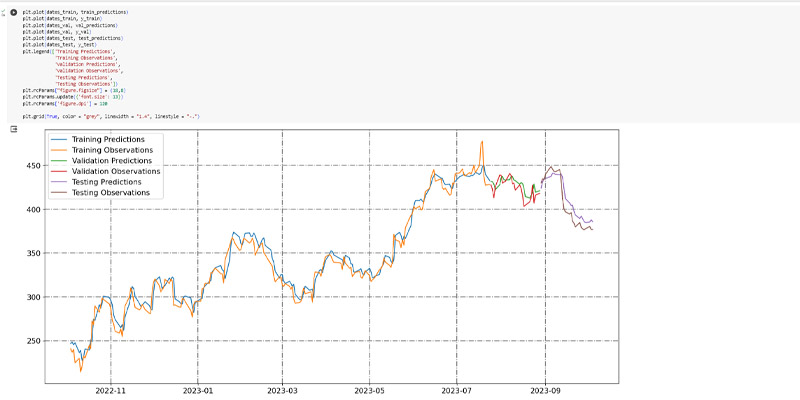
Training Predictions, Training Observations, Validation Predictions, Validation Observations, Testing Predictions, and Testing Observations are combined and plotted on a single chart.
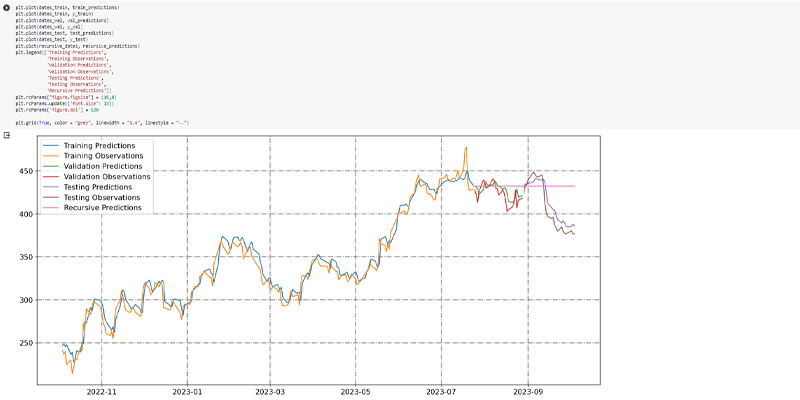
It’s important to note that predicting stock prices accurately is inherently challenging due to the unpredictable nature of financial markets. Factors such as market sentiment, geopolitical events, and unexpected news can significantly impact stock prices, making it difficult for any model, including LSTM, to provide perfectly precise predictions. Therefore, while LSTM models offer powerful tools for analysis, they should be used in conjunction with other methods and by experienced professionals who understand the limitations and risks associated with stock market predictions.
The reliability of LSTM models, or any machine learning model, for prediction depends on various factors, including the quality of the data, the features used for prediction, the model architecture, and the expertise of the data scientists or researchers developing the model. LSTM models are particularly well-suited for time series prediction tasks, but they are not a magic solution and have limitations.
Financial markets are complex, influenced by a wide range of factors including economic indicators, political events, market sentiment, and many others. LSTM models, while powerful, might not always capture the subtle nuances and sudden shifts in these factors. Therefore, it’s crucial to be cautious when using LSTM models or any predictive models for financial predictions. They should be seen as tools to aid decision-making rather than crystal balls that provide infallible predictions. It’s always a good practice to combine machine learning models with expert financial analysis and to consider multiple sources of information for making important financial decisions.
Disclaimer for Data Analysis and Data Science
** The purpose of creating this Data Analysis and Data Science is solely for educational use, and any commercial use is strictly prohibited.
This disclaimer (“Disclaimer”) is provided to inform users of the data analysis and data science findings (“Analysis”) that the following terms and conditions govern its use. By accessing and using the Analysis, you acknowledge and agree to the terms set forth herein. If you do not agree with these terms, please do not use the Analysis.
1. Purpose and Nature of the Analysis
The Analysis is intended exclusively for informational and analytical purposes. It is not intended as professional advice, and it should not be considered as a substitute for expert consultation or guidance in specific areas of expertise. The Analysis is a representation of data at a particular point in time and should not be interpreted as a definitive source of information.
2. Data Accuracy and Limitations
The data used in the Analysis is sourced from various publicly available or authorized data sets and may be subject to inaccuracies, errors, or omissions. Users are advised to independently verify any critical information or data points presented in the Analysis. The Analysis may also involve assumptions and simplifications that can impact the accuracy and comprehensiveness of the results.
The results of this analysis may be subject to limitations, assumptions, and uncertainties inherent to the data sources, methods, and models employed. Any decisions or actions taken based on the information in this analysis are the sole responsibility of the user, and we disclaim any liability for the consequences of such decisions or actions.
3. User Responsibility
Any decisions, actions, or interpretations made based on the Analysis are solely the responsibility of the user. We disclaim any liability for the consequences of such decisions or actions. Users should exercise caution and critical judgment when utilizing the Analysis to inform any course of action.
4. Data Privacy and Ethical Considerations
Users must respect data privacy and ethical principles when conducting their own analyses or using information presented in the Analysis. Ensure that you have the necessary permissions and adhere to all applicable laws and regulations when handling and analyzing data, particularly when working with sensitive or personal data.
5. No Warranty
We make no warranties or representations regarding the accuracy, completeness, or suitability of the Analysis for any specific purpose. The Analysis is provided “as is,” and we expressly disclaim all warranties, express or implied, including but not limited to the implied warranties of merchantability and fitness for a particular purpose.
6. Changes and Updates
This Disclaimer may be subject to change without notice. Users are encouraged to review it periodically for any updates or modifications. Continued use of the Analysis following changes to this Disclaimer implies acceptance of the revised terms and conditions.
7. Contact Information
If you have any questions, concerns, or require clarification about this Disclaimer or the Analysis presented herein, please seek professional advice or contact us directly at [Contact Information].
This detailed disclaimer provides users with a comprehensive understanding of the limitations, responsibilities, and purpose of the data analysis, emphasizing the importance of independent verification, ethical considerations, and user discretion.